TL;DR:
- AI is reshaping modern banking by automating key functions across front, middle, and back-office operations, enhancing efficiency, accuracy, and innovation.
- AI-driven automation is enhancing banking processes in areas like finance, risk and compliance, acquisition management, HR, IT, and product development, reducing costs and improving decision-making.
- Robotic Process Automation (RPA), Machine Learning (ML), and AI tools streamline processes such as customer onboarding, fraud detection, credit scoring, and regulatory compliance, improving operational efficiency and decision accuracy.
- Despite the benefits, AI integration poses challenges related to data quality, transparency, regulatory compliance, bias, and legacy system integration.
- AI enables banks to deliver personalized customer experiences, optimize workflows, and maintain a competitive edge.
In 2016, AlphaGo, an AI system, defeated 18-time world champion Lee Sedol in Go, a complex board game known for requiring intuition, creativity, and strategic thinking—skills once thought to be uniquely human. Since then, AI technologies have continued to advance rapidly, with their transformative effects becoming increasingly visible across various industries. AI-driven systems are now personalizing digital content recommendations based on individual tastes, creating clothing designs for fashion retailers, and even surpassing seasoned doctors in identifying early signs of cancer. For global banking, McKinsey estimates that AI technologies could deliver up to $1 trillion of additional value annually.
As consumers increase their use of digital banking services, they expect more, particularly compared to the standards they are accustomed to from leading consumer internet companies. Meanwhile, these digital experience leaders continuously raise the bar on personalization to the point where they sometimes anticipate customer needs before they are aware of them and offer highly tailored services at the right time through the right channel. Banking automation can be that channel.
Banking Automation
Banking automation has become a crucial component in the modernization of financial services. As the demand for digital, faster, and more efficient banking grows, automation helps institutions streamline operations, enhance customer experiences, and reduce costs.
The leading innovators in the industry are already making the step from AI as an 'instrument of strategy' (i.e., accelerating delivery of today's business plan) to a 'determinant of strategy', where tomorrow's business is planned around new AI capabilities. JP Morgan Chase sees the transformational impact that AI can have and plans to spend $1B or more a year on AI capabilities.
Banking Segments Improved by Automation
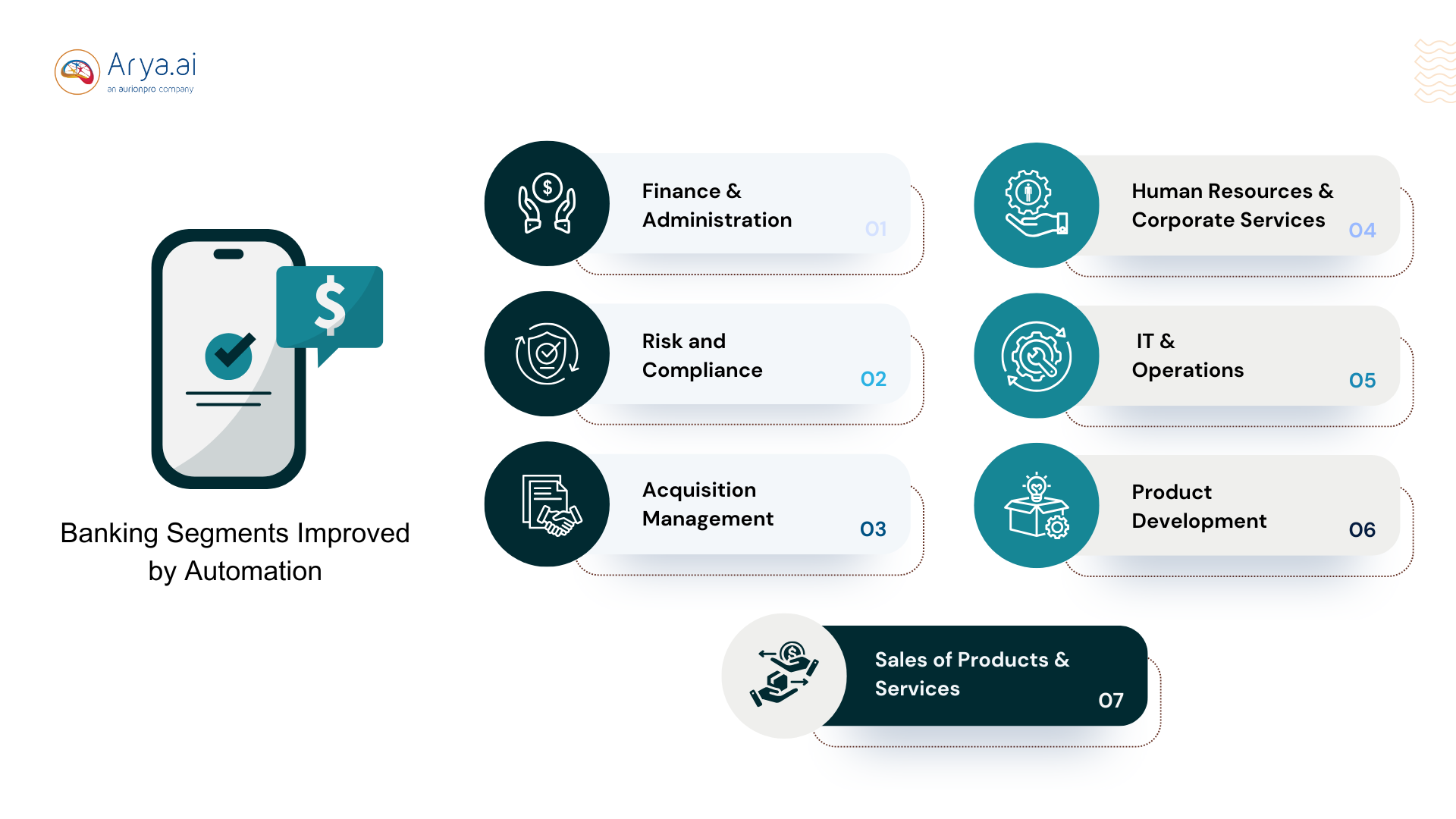
1. Finance & Administration
Automation is transforming finance departments within banks by streamlining processes such as budgeting, forecasting, and financial reporting. Robotic Process Automation (RPA) automates routine tasks like data reconciliation, invoice processing, and financial statement preparation.
Key Impacts:
- Reduction in manual errors and time spent on repetitive tasks.
- Enhanced real-time reporting and financial analysis.
- Cost savings through efficient administrative operations.
2. Risk and Compliance
Automation is a game-changer in risk management and regulatory compliance. AI and machine learning (ML) algorithms monitor real-time transactions, detecting potential fraud, money laundering, and other suspicious activities.
Key Impacts:
- AI-driven fraud detection and prevention systems that minimize financial crime.
- Streamlined compliance management through automated reporting and audit trails.
- Real-time monitoring and early detection of risk exposure.
3. Acquisition Management
Customer acquisition is being transformed by automation through the use of AI to personalize marketing campaigns, predict customer behavior, and optimize lead generation.
Key Impacts:
- Increased customer acquisition through personalized and automated campaigns.
- Improved lead conversion using predictive analytics.
- Optimized marketing spending through data-driven insights.
4. Human Resources & Corporate Services
HR and corporate services in banking benefit from automation in areas such as recruitment, payroll processing, employee onboarding, and performance management.
Key Impacts:
- Faster recruitment and onboarding through AI-powered tools.
- Reduced administrative workload in HR and corporate services.
- Better employee engagement with automated HR solutions (e.g., self-service portals).
5. IT & Operations
Automated workflows improve service delivery by ensuring minimal downtime, while AI-driven systems detect anomalies in real time to prevent cybersecurity threats.
Key Impacts:
- Increased operational efficiency through automated workflows.
- Enhanced cybersecurity with AI-driven threat detection.
- Reduced system downtime and improved infrastructure scalability.
6. Product Development
Automation enables faster product development using AI to analyze customer needs, market trends, and competitive offerings helping banks create personalized financial products.
Key Impacts:
- Faster time-to-market for financial products.
- Improved product customization using customer data and AI.
- Data-driven decision-making in product development and enhancements.
7. Sales of Products & Services
AI algorithms assist in identifying potential customers, personalizing offers, and automating loan approval processes.
Key Impacts:
- Automated loan processing and approval, reducing turnaround times.
- Increased cross-selling opportunities through personalized, data-driven offers.
- Enhanced customer service with AI chatbots providing instant product information.
Key Technologies Driving Banking Automation
Robotic Process Automation (RPA):
RPA allows banks to streamline processes such as data entry, transaction processing, and report generation, all without human intervention. This leads to significant cost reductions, fewer errors, and enhanced operational efficiency. Banks use RPA to automate back-office functions like reconciliation, compliance checks, and customer onboarding.
Machine Learning (ML):
ML models analyze vast datasets to detect patterns, predict customer behavior, and identify risks such as fraudulent transactions. In areas like credit scoring, fraud detection, and predictive analytics, ML enables banks to make more informed decisions faster and more accurately.
Artificial Intelligence (AI):
AI-powered tools such as chatbots and virtual assistants can handle customer service queries around the clock, providing instant support and personalized responses. In risk management, AI can analyze vast amounts of transaction data, accurately identifying potential fraud or compliance issues.
How AI is Reshaping Banking Workflows
Artificial Intelligence (AI) is revolutionizing banking by automating key functions across all operational tiers—front office, middle office, and back office. In an era of heightened customer expectations, complex regulatory demands, and increasing competition, AI provides banks with the tools to enhance the overall customer experience. This tiered approach ensures that AI impacts every aspect of banking, driving efficiency, accuracy, and innovation across the board.
Tier 1: Front Office (Customer Facing Services)
Enhancing Customer Service with AI:
AI-powered chatbots and virtual assistants are revolutionizing customer service by automating routine interactions. These AI systems can handle various tasks, such as answering frequently asked questions, processing simple transactions, and even providing personalized financial advice. According to McKinsey, automation can reduce operational costs in banks by up to 30%, particularly in areas like transaction processing, customer experience, and compliance.
Personalized Banking Experiences:
Banks that adopt a customer-centric approach leverage data and technology to offer personalized services tailored to individual customer preferences. AI and data analytics help banks analyze customer behavior, spending patterns, and financial goals, enabling them to offer customized product recommendations, relevant financial advice, and targeted offers.
Tier 2: Middle Office Operations (Risk and Compliance Management)
Improving Risk Management and Fraud Detection:
Machine learning algorithms can analyze massive datasets to identify suspicious transaction patterns and flag them immediately. Banks like Citibank have slashed phishing attacks by 70% thanks to ML. AI can flag unusual spending behaviour or detect anomalies in transaction histories, allowing banks to respond promptly and mitigate potential losses.
Regulatory Compliance:
Artificial Intelligence (AI) is playing a transformative role in enhancing banking regulatory compliance by automating complex processes, improving accuracy, and ensuring that banks adhere to the ever-evolving regulatory landscape.
- Anti-Money Laundering (AML) and Fraud Detection: AI uses machine learning to detect suspicious transactions in real time, improving fraud detection and reducing false positives.
- Know Your Customer (KYC) Compliance: AI automates customer identity verification, document extraction, and risk assessment, streamlining KYC processes and ensuring ongoing monitoring.
- Data Privacy and Protection: AI helps banks comply with data privacy regulations (like GDPR and CCPA) by automating data classification, encryption, and access monitoring.
- Regulatory Reporting and Audit Trails: AI automates regulatory reporting, ensuring compliance with documentation standards and reducing errors and delays.
- Monitoring Regulatory Changes: AI continuously tracks changes in financial regulations, alerting banks to new requirements and enabling them to stay compliant.
Tier 3: Back Office Operations
Automating Back-office Operations and Decision Making:
Beyond customer-facing functions, AI is also streamlining back-office operations, improving efficiency in loan underwriting, credit scoring, and compliance monitoring. In compliance, AI systems can automatically scan transactions for compliance with regulatory requirements, flagging any issues that need attention.
AI in Transaction Reconciliation:
AI automates transaction reconciliation by quickly matching financial records across various systems, identifying discrepancies, and resolving them with minimal human intervention. Machine learning algorithms analyze transaction data, detect patterns, and flag inconsistencies.
Loan Processing:
The technology has streamlined document verification, speeds up decision-making, and reduces the time required to approve or reject loans. Some banks have reported reducing loan processing time to as low as 30 to 60 seconds using AI-powered algorithms.
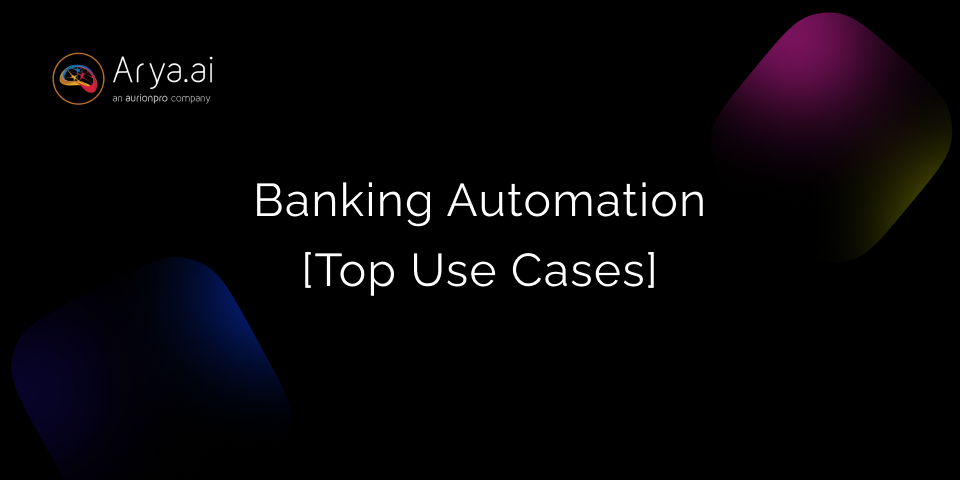
Challenges AI Integration Poses in the Banking Industry
While AI offers immense benefits, its integration into the banking sector comes with significant challenges. These hurdles range from technical and operational complexities to ethical and regulatory concerns. Let's explore some key challenges and how banks can overcome them.
1. Data Quality and Availability
AI models rely heavily on high-quality data to deliver accurate insights and predictions. However, banks often struggle with fragmented, incomplete, or outdated data spread across different systems and departments.
Solution:
Banks must invest in data management infrastructure that consolidates data from multiple sources into a unified, clean, and structured format. Implementing data governance frameworks and ensuring regular data audits will help maintain data integrity.
2. Lack of Transparency and Explainability
AI models, particularly complex ones like deep learning, are often seen as "black boxes" due to their lack of transparency. This poses a challenge in banking, where transparency and accountability are critical, especially for tasks like loan approvals or fraud detection.
Solution:
To address this, banks can implement Explainable AI (XAI) techniques, which provide understandable justifications for AI decisions. Banks should also prioritize using models that balance performance with explainability, ensuring stakeholders can trust AI-driven insights.
3. Regulatory and Compliance Issues
AI in banking must comply with stringent regulatory frameworks that govern data privacy, security, and financial transparency. Regulations like the GDPR (General Data Protection Regulation) and CCPA (California Consumer Privacy Act) require banks to protect customer data and explain AI-driven decisions.
Solution:
Banks must engage compliance teams early in AI development and ensure that AI models align with regulatory requirements. Working closely with regulators to establish AI guidelines and best practices will also aid in the smoother adoption of AI technologies while adhering to legal standards.
4. Bias and Fairness Concerns
AI models, if not carefully designed, can perpetuate biases in decision-making, leading to unfair treatment of customers.
Solution:
Regularly auditing AI models for discriminatory outcomes is crucial to identify and correct biases. Banks can also apply fairness constraints to AI models, ensuring that decisions are made equitably across different customer demographics.
5. Integration with Legacy Systems
Many banking institutions operate on legacy systems that are not built with AI integration. Merging cutting-edge AI technologies with older, rigid infrastructures poses a challenge, as legacy systems often lack the flexibility to support advanced AI tools.
Solution:
Banks can adopt a phased approach to AI integration by gradually modernizing their legacy systems through APIs and cloud-based platforms. Implementing middleware solutions can help bridge the gap between legacy systems and AI technologies.
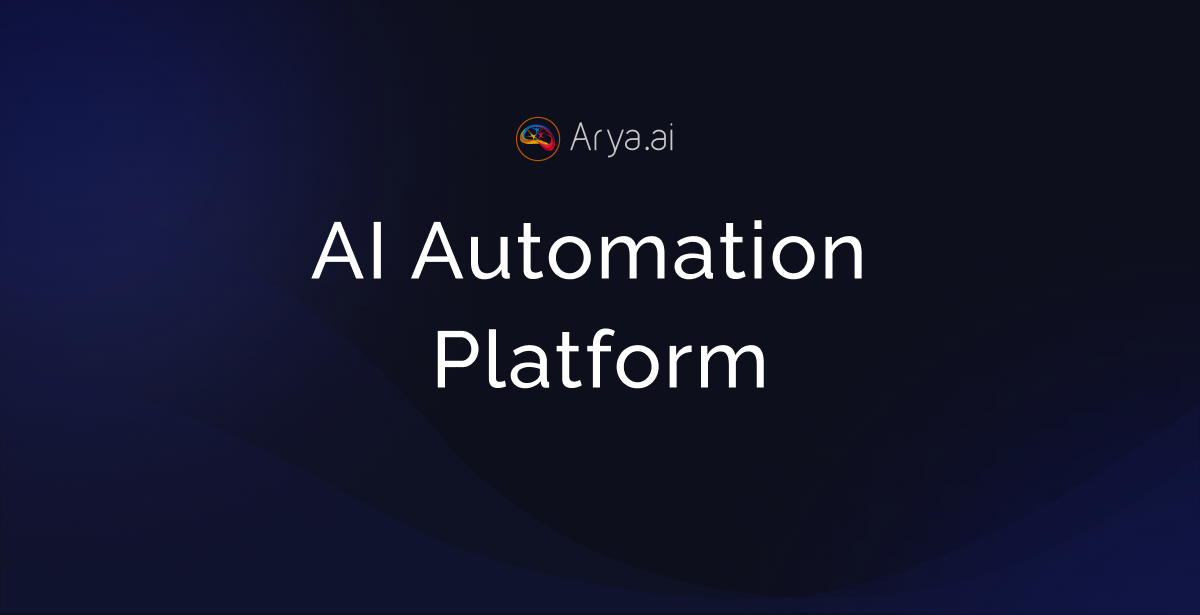
Conclusion
AI is not just a tool for improving efficiency—it is a transformative force that is redefining the future of banking. As banks face increasing competition, evolving customer expectations, and tighter regulatory scrutiny, AI-driven automation provides the agility and intelligence needed to stay ahead.
However, successful AI integration requires thoughtful implementation, addressing challenges like data quality, regulatory compliance, and bias. Banks must focus on building robust AI frameworks that prioritize transparency, fairness, and security while fostering a culture of continuous innovation.
The future of banking lies in leveraging AI's potential to optimize processes, deliver personalized experiences, and remain competitive in an ever-changing financial landscape. Banks that embrace this shift today will be better equipped to lead tomorrow.