AI has been the buzzword for the past couple of years. Businesses across sectors and different sizes are trying their hand at AI technologies to stay competitive. The BFSI industry, in particular, is witnessing an AI-powered transformation that benefits both organisations and customers.
The global AI market in BFSI is estimated to grow at a CAGR of 32.5% from 2024 to 2031. However, in this transformative wave, while investors and stockholders are jumping on the AI bandwagon, few are aware of the distinct kinds of AI that are driving the future of BFSI - Generative AI and Predictive AI.
While the former excels in areas such as fraud detection, automated report generation and personalized customer interactions, the latter flexes its muscles in analyzing historical data to forecast future trends, making it invaluable for risk assessment, credit scoring and market trend analysis.
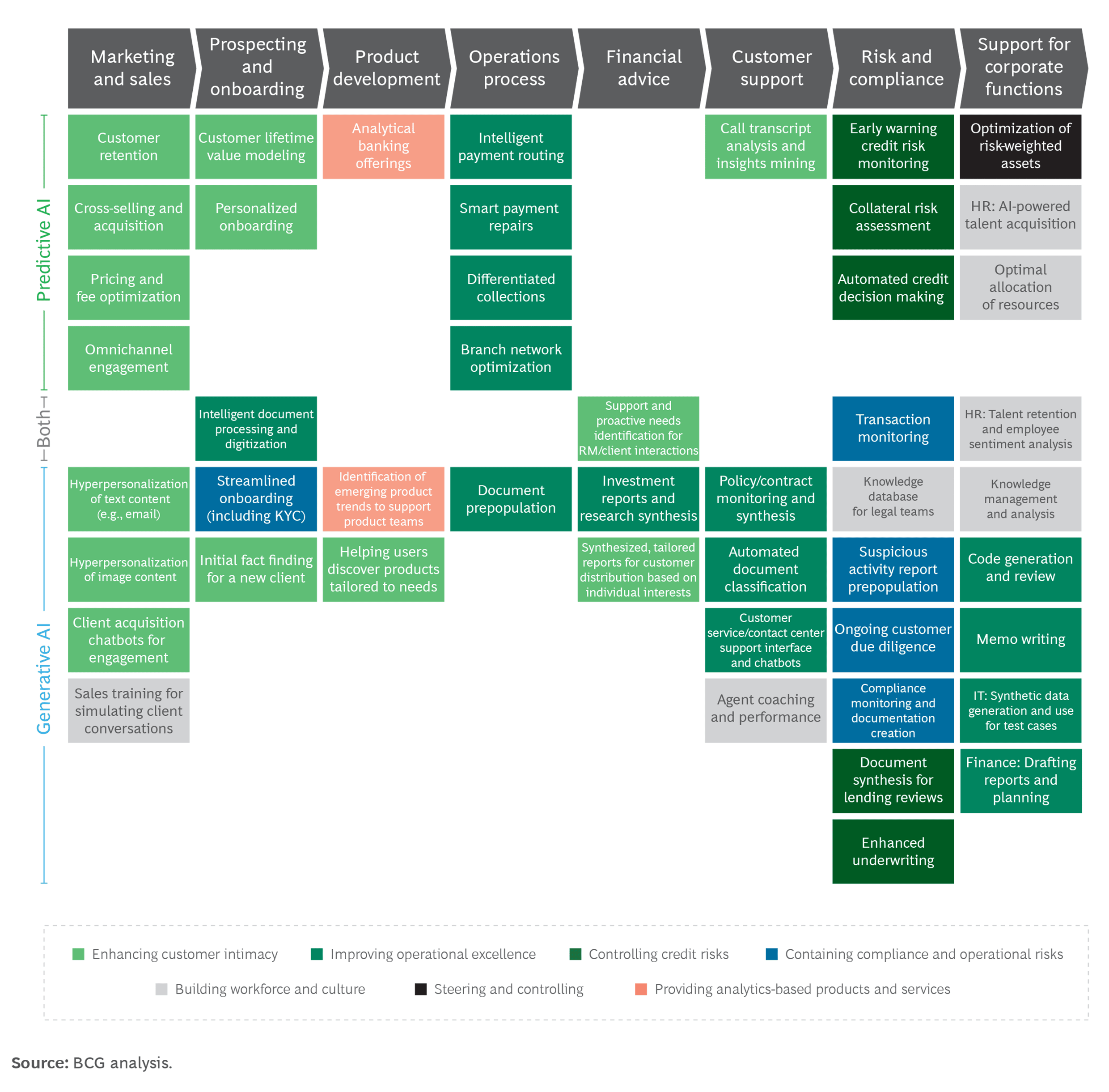
This blog post explores the distinct characteristics, applications, and potential benefits of Generative AI and Predictive AI, specifically focusing on their relevance and impact within the BFSI sector.
What is Generative AI?
Generative AI or GenAI, is a subset of Artificial Intelligence (AI) technologies that centers around creating new content, solutions and data patterns. While traditional AI systems analyse existing data and patterns for insights and predictions, GenAI generates novel outputs by learning from large data sets.
These outputs can range from text, images, music and video to complex data structures, making it one of the most powerful technologies to date. To put it simply, instead of just replicating or predicting content based on existing data, Generative AI learns the underlying structures and patterns to produce “original” content.
How Generative AI Works?
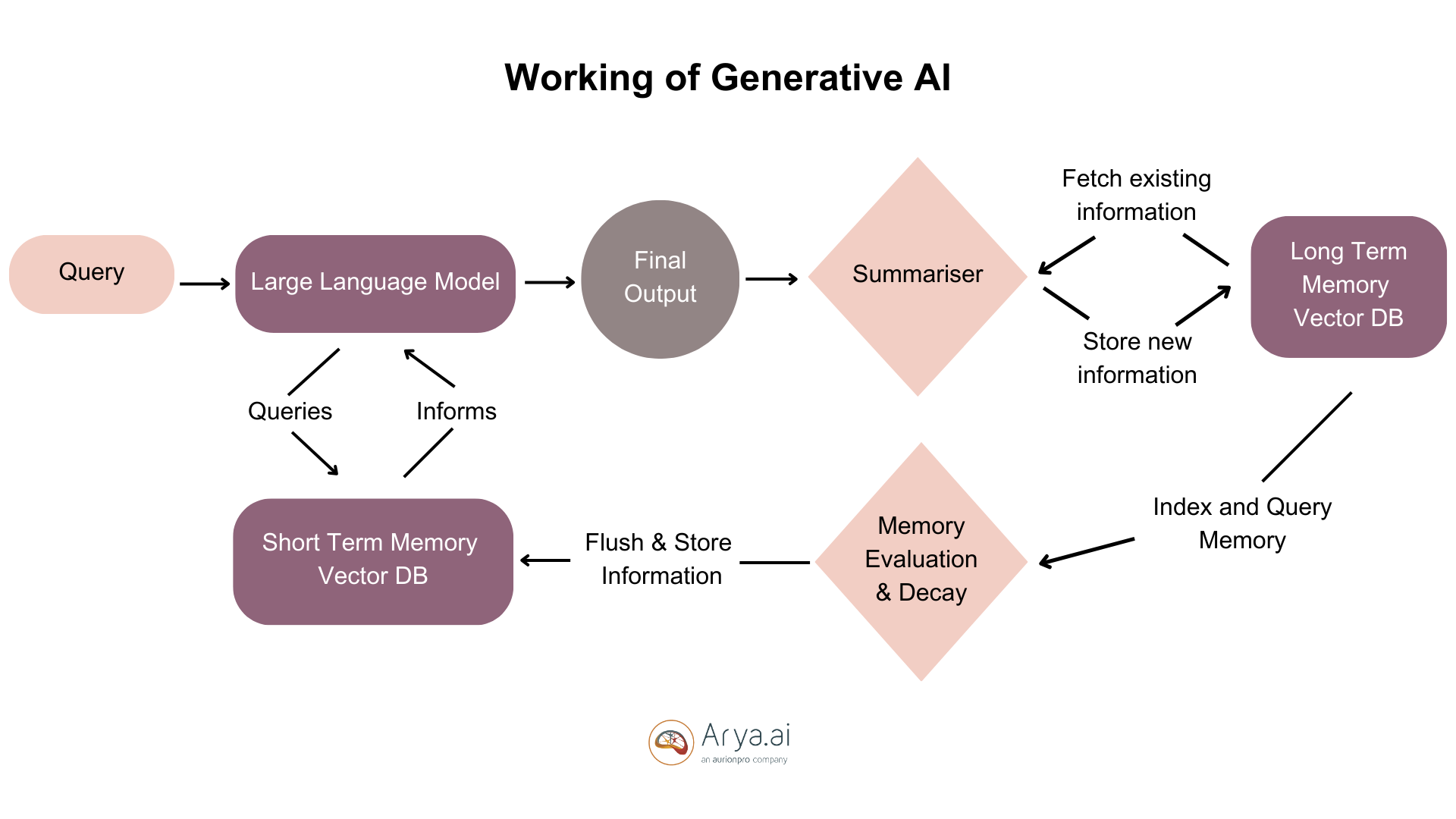
At the centre of GenAI are neural networks. Neural networks are complex algorithms, inspired by the structure and function of the human brain. These networks have different nodes connected to each other that process and transmit information.
GenAI uses Machine Learning (ML) algorithms to scour large datasets and distinguish patterns and structures within the data. This allows the model to observe the basic rules that govern the creation of the content it has been trained on.
Generative AI models are commonly trained using two methods:
1. Generative Adversarial Networks (GANs)
GANs have two distinct neural networks that are pitted against each other in a competitive learning process. These are the generator and the discriminator.
- Generator: This network creates new data instances, with the goal of imitating the patterns of the training data.
- Discriminator: This network evaluates the generated data against real data and provides feedback to the generator.
Through this opposing process, both networks improve their performance. The generator becomes better at creating realistic data and the discriminator becomes more adept at detecting synthetic data. This iterative training continues until the generator produces data that the discriminator can no longer distinguish from real data.
2. Variational Autoencoders (VAEs)
Variational Autoencoders (VAEs) are generative models that have two neural networks: the encoder and the decoder. Here’s how they work:
- Encoder: When given input data, the encoder compresses it into a simplified representation stored in a “latent space.” This condensed version retains important information needed by the decoder.
- Decoder: The decoder reconstructs the original data from the latent representation. It collaborates with the encoder to learn an efficient and streamlined data representation.
VAEs allow users to sample new latent representations easily. The decoder can then map these representations to generate novel data. While VAEs can quickly generate outputs like images, their level of detail may not match that of diffusion models like GAN.
An important factor influencing the development of generative models is the architecture underneath the neural network. A popular and highly effective architecture is the transformer network.
Transformer networks excel at processing sequential data, like text, by using a mechanism called Attention. This mechanism weighs the importance of different parts of the input text when making predictions. ChatGPT or Chat Generative Pre-trained Transformer is an example.
Applications of Generative AI in BFSI
Generative AI has some innovative applications in the BFSI sector that improve efficiency in the organization and give better customer experiences. Here are some key areas:
- Fraud Detection and Prevention:
Generative AI can create synthetic data that mimics fraudulent transactions. This helps financial institutions train their fraud detection systems more effectively. By exposing these systems to a wider variety of fraudulent patterns, they become more adept at finding and reducing real-world fraud.
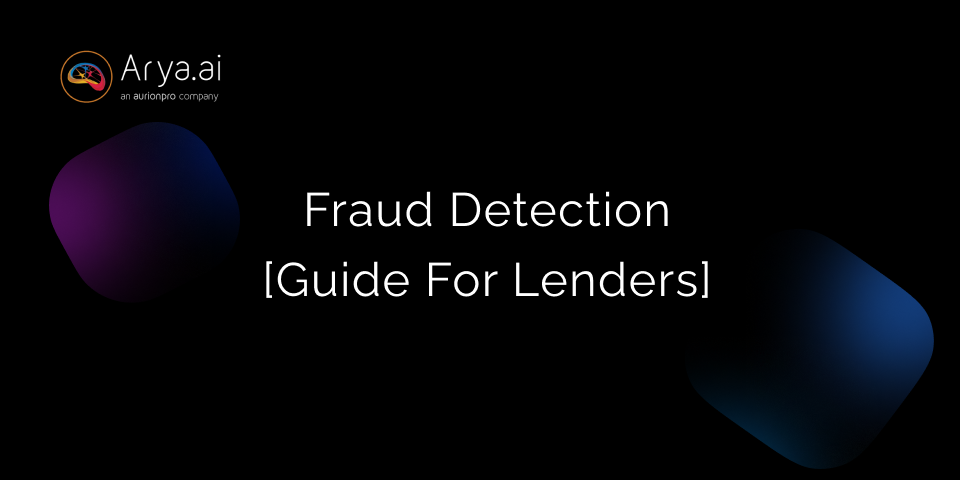
- Automated Report Generation:
Financial institutions generate vast amounts of reports, ranging from compliance documents to market analysis. Generative AI automates this process by creating detailed and accurate reports, saving time and reducing the risk of human error.
- Personalization of Customer Interactions:
The BFSI sector is immensely competitive. So personalized customer experiences are crucial for the customers. Generative AI enables organisations to create tailored content and product recommendations by analyzing customer behaviour and preferences. For instance, chatbots powered by Generative AI can engage customers in natural, human-like conversations.
Real-world Examples of Generative AI in BFSI
- JPMorgan Chase used a Generative AI system named COiN (Contract Intelligence) way back in 2017, to automate the review of legal documents and extract critical data points. This system has saved the bank over 360,000 hours of manpower annually.
- A more recent example was at HSBC, where the organisation collaborated with Google Cloud to develop an AI solution, named Anti Money Laundering AI (AML AI). This significantly improved the detection of suspicious financial activities, reduced false positives and enhanced the precision of identifying potential money laundering.
What is Predictive AI?
Predictive AI, more commonly known as Predictive Analytics, is a form of AI that predicts future events or trends using historical data, statistical algorithms, machine learning models, and data mining techniques. The goal is to find patterns and relationships within data to help businesses make informed decisions.
Predictive AI depends on large data sets to make predictions, and so its effectiveness relies heavily on how accurate and vast the data is. It differs from traditional business intelligence by going beyond logical data analysis to forecast future events and trends. Instead of absolute certainty, predictive analytics provides educated guesses based on patterns in the data.
How Predictive AI Works?
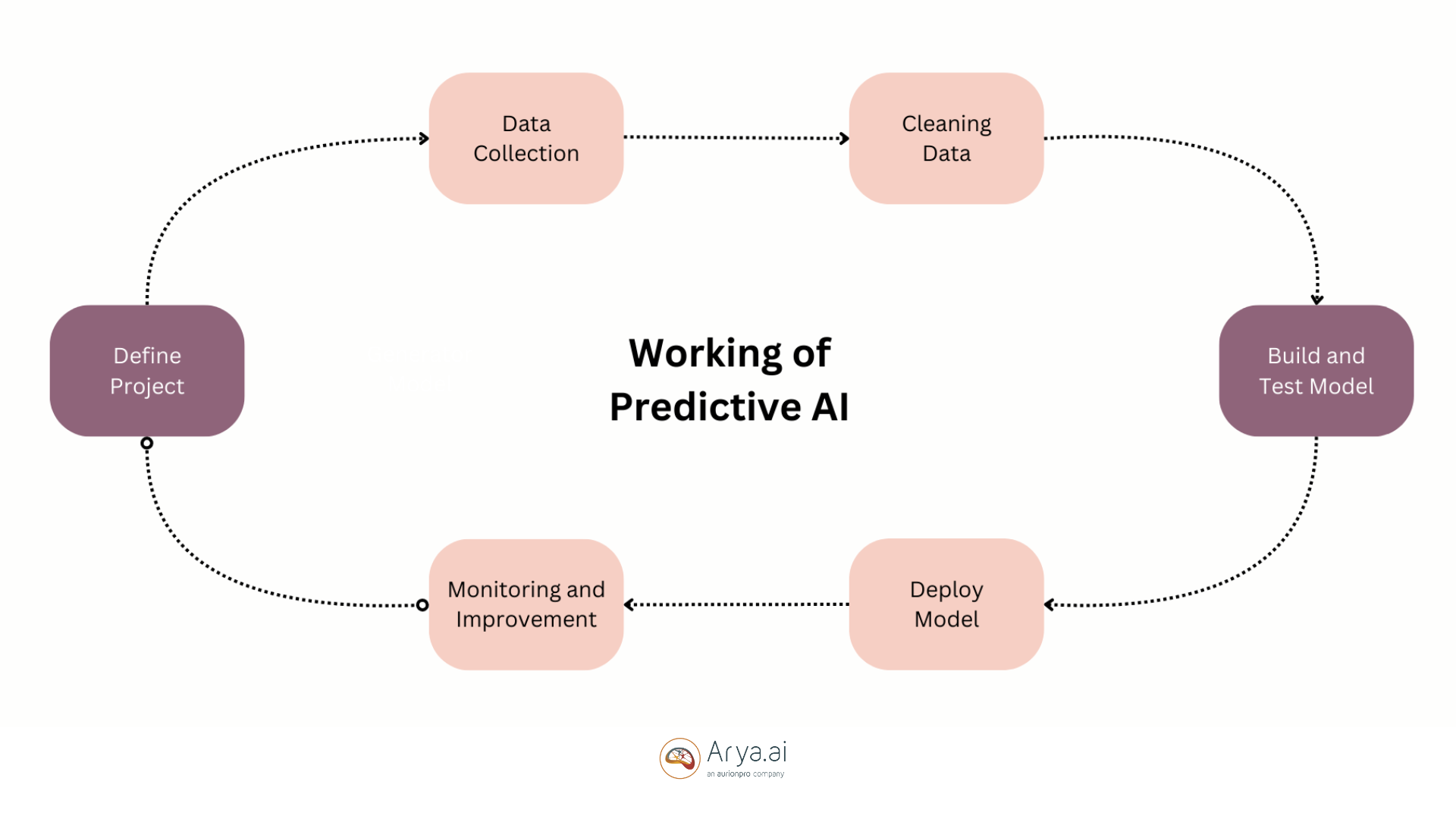
Predictive AI works through a set of systematic processes that use statistical models and machine learning algorithms. Here’s a step-by-step breakdown:
1. Data Collection
The process begins with gathering relevant data from all kinds of sources, like databases, sensors, transaction records, customer interactions, market trends, and social media. All this data may be either structured (like Excel sheets), or unstructured (like text, images, or audio).
2. Data Preprocessing
Once the data is collected, it undergoes preprocessing to prepare it for analysis. Preprocessing steps include:
- Data Transformation: Converting raw data into a usable format.
- Feature Engineering: Identifying and creating relevant features (variables).
- Data Splitting: Dividing data into training and testing sets to evaluate the model’s performance.
3. Model Selection and Training
The core of Predictive AI lies in the machine learning models used to analyze the data. There are various algorithms available, each suited for different types of prediction tasks:
- Regression Models: For predicting continuous values (e.g., stock prices).
- Classification Models: For categorizing data (e.g., detecting fraud).
- Time Series Models: For making predictions over time (e.g., sales forecasting).
- Ensemble Models: Combining multiple models for better accuracy.
The chosen model is then trained using the training data, learning patterns and relationships to make predictions.
4. Model Evaluation
After training, the model is evaluated on the test dataset to assess its accuracy and robustness. Key metrics used for evaluation include:
- Accuracy: How often the model’s predictions are correct.
- Precision and Recall: Precision measures the accuracy of positive predictions, while recall measures the ability of the model to identify all positive instances.
- F1 Score: A harmonic mean of precision and recall, giving a single metric to evaluate the model’s performance.
5. Prediction and Deployment
Once validated, the model is used to make predictions on new data. This can be done in real-time (e.g., predicting loan default risk during application) or in batches (e.g., forecasting quarterly sales).
6. Continuous Monitoring and Improvement
The model is continuously monitored to ensure it remains accurate. It’s periodically retrained with new data to keep up with changing patterns.
Applications of Predictive AI in BFSI
Predictive AI has now become an indispensable tool in the BFSI sector. Key applications include:
- Risk Assessment and Management
By analyzing historical data on loans, investments, and market trends, predictive analytics models can predict the likelihood of default, market fluctuations, and other risk factors. This helps banks and insurance companies to make data-driven decisions, curb potential losses, and improve risk management strategies.
- Credit Scoring and Loan Approval
Traditional credit scoring models rely on static data points and can be limited in their predictive power. Predictive AI enhances credit scoring by adding a wider range of variables, including transaction history, social media behaviour, and other non-traditional data sources. This results in more accurate credit assessments and faster loan approval processes.
- Market Trend Analysis and Forecasting
Predictive AI models analyze historical market data, news, social media sentiment, and other relevant information to forecast market trends. These predictions help financial institutions and investors make better decisions, optimize trading strategies, and capitalize on market opportunities.
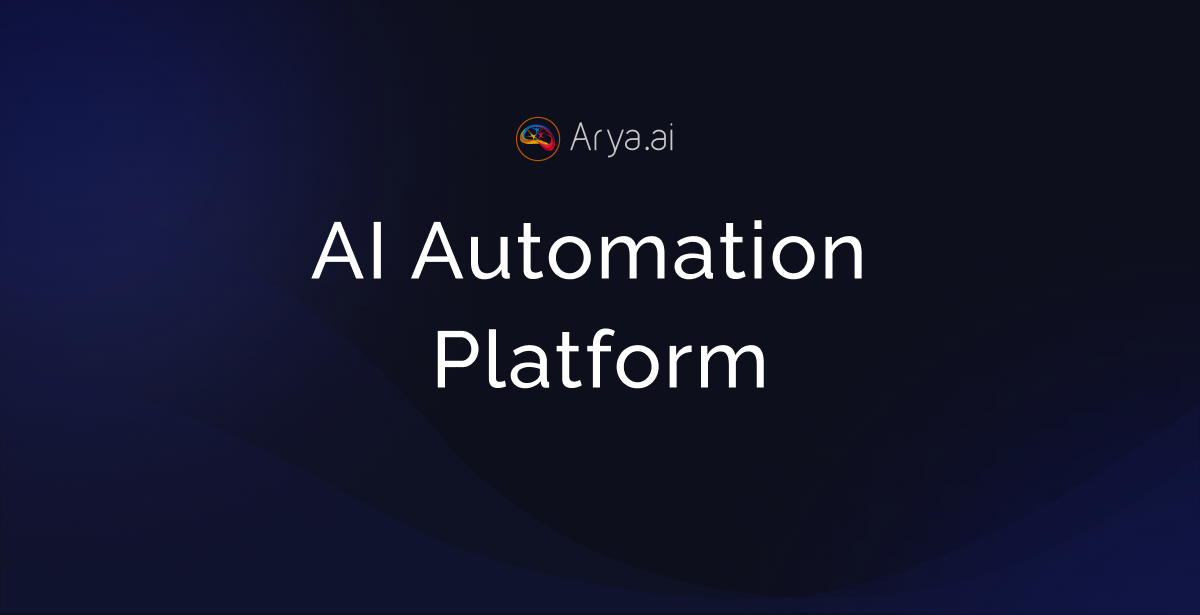
Real-world Examples of Predictive AI in BFSI
- Bank of America has adopted Predictive AI to predict the likelihood of companies defaulting on loans. They use natural language processing (NLP) to analyze corporate credit risk. By digesting earnings-call transcripts, the AI estimates companies’ probability of default over the next 12 months.
- UBS, a prominent financial institution, integrated PredictX’s artificial intelligence and predictive analytics to enhance its travel and expense management. The goal was to use these tools to develop more accurate corporate travel budgets and forecasts that take seasonal changes into account.
Key Differences Between Generative AI and Predictive AI
Understanding the differences between Generative AI and Predictive AI is essential for effectively integrating these technologies into your organization's workflow.
Generative AI focuses on creating new, synthetic data or content, using models to generate realistic data samples, such as synthetic customer data for training fraud detection systems or personalized communication scripts. It requires extensive and diverse datasets and involves high complexity with significant computational resources and expertise.
Predictive AI, on the other hand, forecasts future outcomes based on historical data by analyzing patterns and trends. It relies on high-quality historical and structured data and is generally easier to implement with standard machine learning techniques, offering quicker deployment.
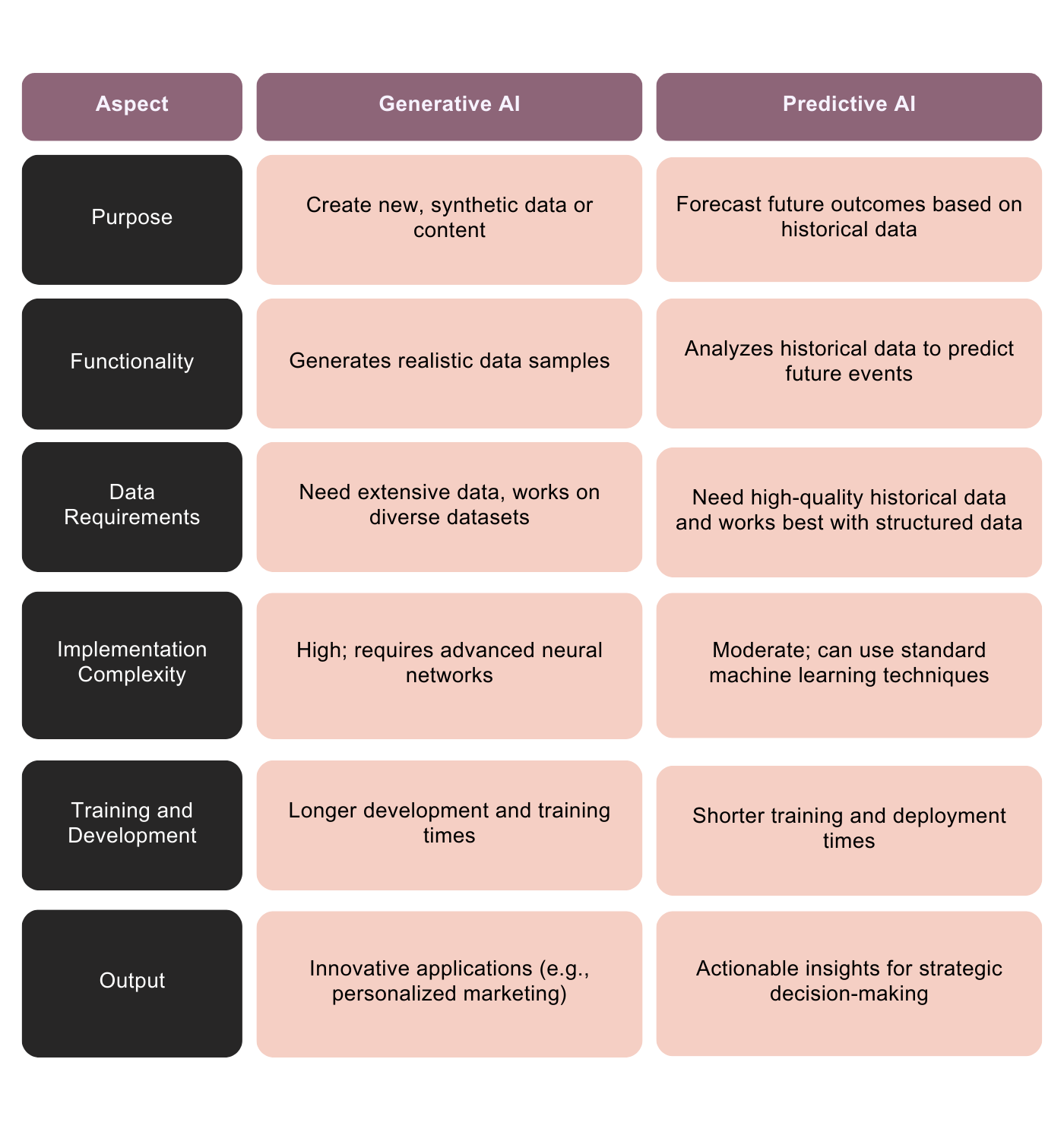
Choosing the Right AI for Your BFSI Business
Choosing the right AI for your BFSI business involves understanding the distinct capabilities of predictive AI and generative AI.
When to choose Generative AI?
- Need for Synthetic Data: If your organization requires large amounts of synthetic data for training, such as enhancing fraud detection systems without compromising real customer data, Generative AI is ideal.
- Personalized Customer Engagement: If personalized customer interactions and content creation are crucial for your business strategy, Generative AI can significantly enhance customer service.
- Innovative Product Development: Use Generative AI when developing new financial products or services that require creativity and innovation.
When to Choose Predictive AI?
- Risk Management: If your primary goal is to improve risk management, Predictive AI’s ability to analyze historical data and forecast future risks is invaluable.
- Data-Driven Decision Making: For businesses looking to make informed decisions based on data analytics, Predictive AI offers actionable insights that can help you plan with a better strategy.
- Operational Efficiency: Choose Predictive AI to streamline processes such as credit scoring, loan approvals, and market trend analysis, to improve efficiency and customer satisfaction.
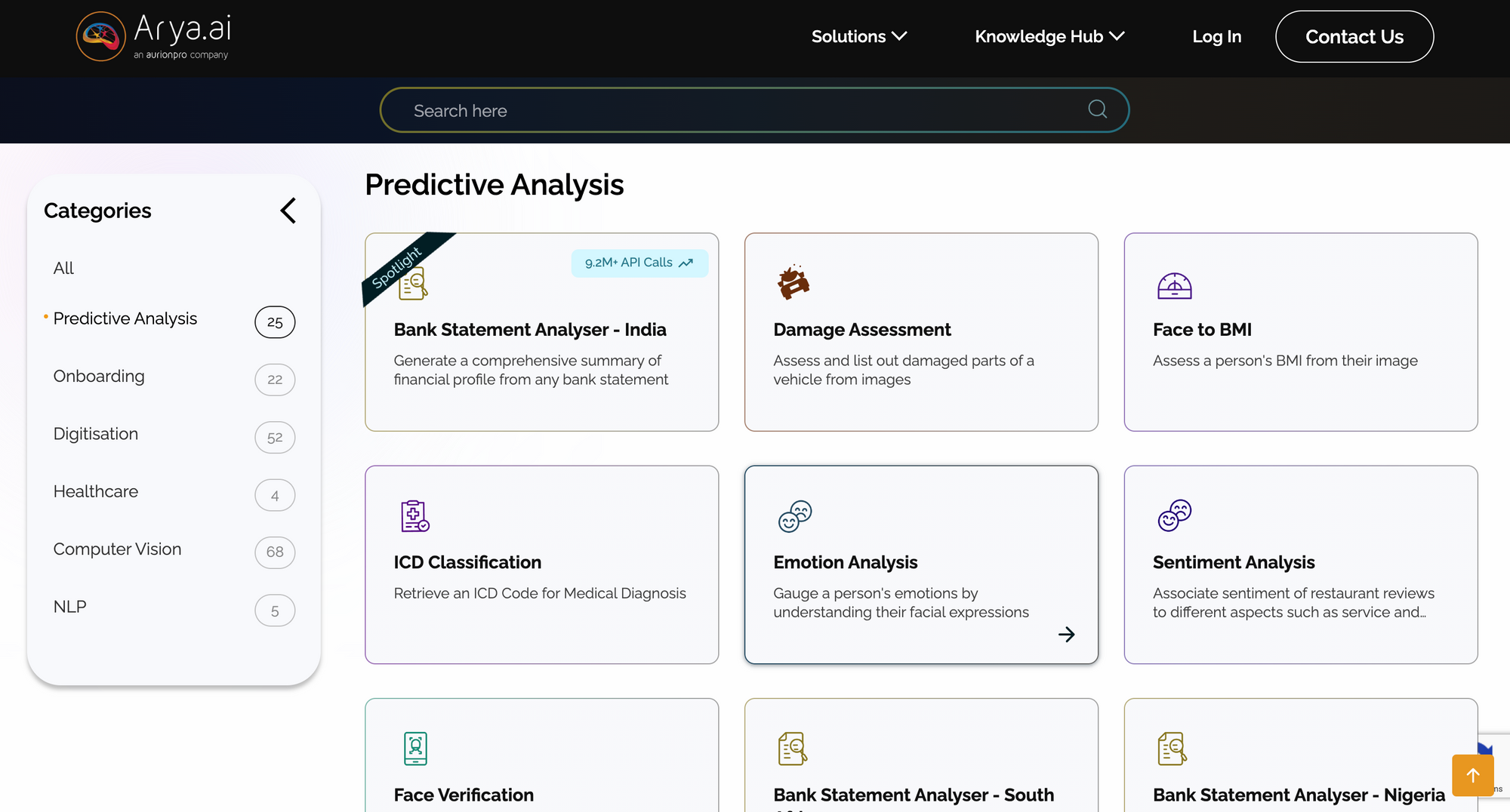
Integrating Both Generative and Predictive AI Technologies
In many cases, the best approach is to integrate both Generative AI and Predictive AI to use their combined strengths.
For example, a BFSI company could use Generative AI to create synthetic training data for fraud detection systems while employing Predictive AI to forecast market trends and manage risks. By combining both technologies, your business can achieve a more comprehensive and robust AI strategy that enhances innovation, operational efficiency, and decision-making capabilities.
How Arya AI Leads the Charge in AI Solutions for the BFSI Sector?
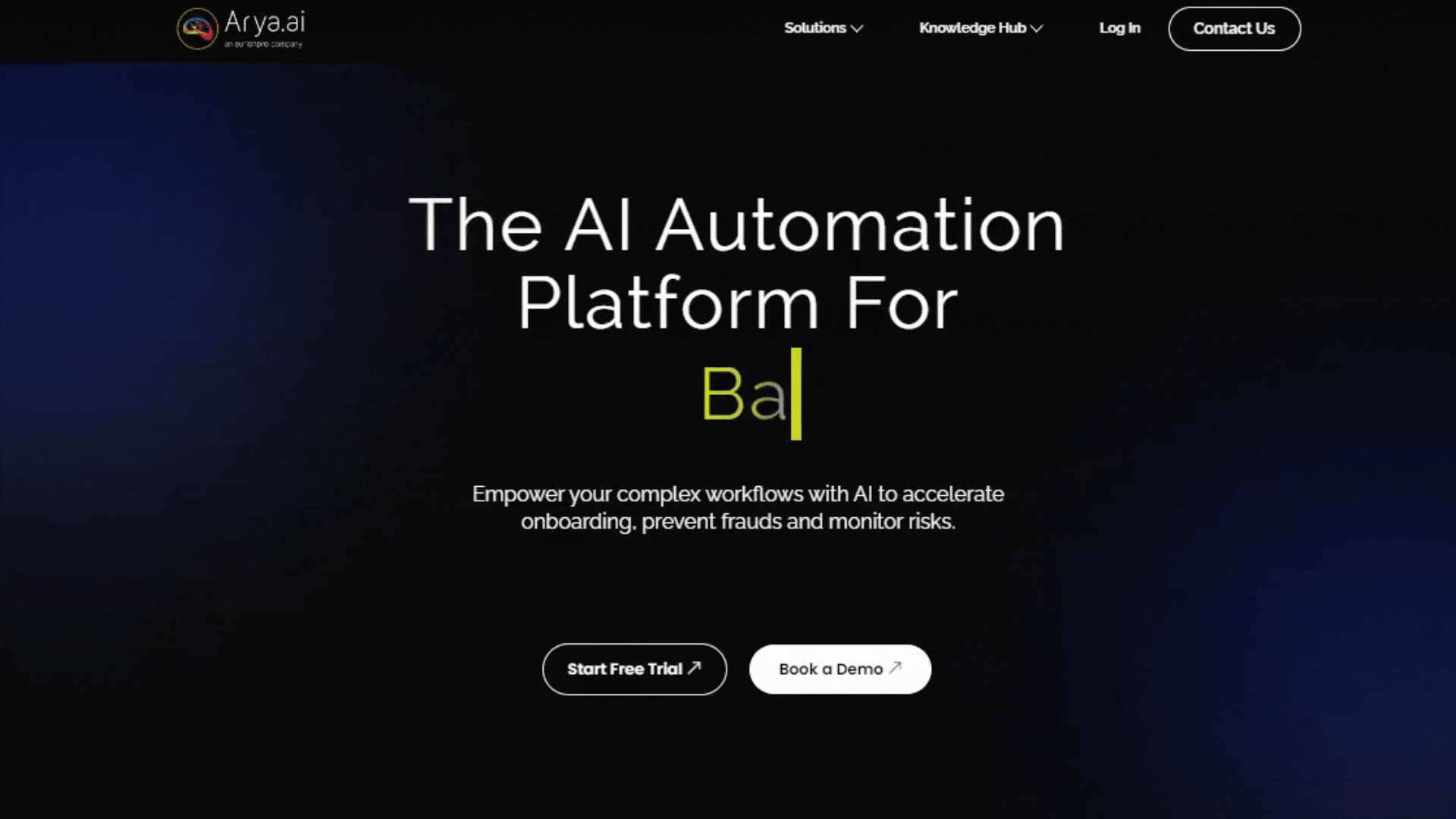
Arya AI is leading the charge for AI adoption in the banking, financial services, and insurance (BFSI) sector in several key ways:
- Pre-Trained AI Solutions: Arya AI has developed a suite of AI-powered solutions tailored explicitly for the BFSI industry, including APIs for Bank Statement Analysis, Document Fraud Detection, KYC Verification, Deepfake Detection and more. This vertical focus allows our app to deliver AI capabilities that directly address the unique needs and challenges of BFSI institutions.
- Vertical-Specific AI Applications: Arya provides AI-powered solutions for BFSI institutions, including automated customer onboarding with document verification, KYC checks, and risk assessment. Additionally, the platform offers AI for critical decision-making and workflow automation, such as cash flow forecasting, and early warning systems for detecting anomalies, fraud, and financial distress.
- Accelerating AI Adoption: Arya AI enables BFSI organizations to rapidly integrate AI into their operations without requiring extensive in-house AI expertise by offering pre-built, task-specific AI models. This lowers the barriers to AI adoption and helps BFSI institutions realize the benefits of AI more quickly.
Conclusion
Choosing between Generative AI and Predictive AI depends on your business's specific needs and objectives, be it in any industry. Generative AI gives you creative solutions and can simulate a wide range of scenarios, making it ideal for marketing, customer engagement, and fraud detection. Predictive AI excels at forecasting future trends and behaviours, giving you valuable insights for risk management, credit scoring, and financial planning.
Arya AI suite of Apps leverages both generative and predictive capabilities, offering custom solutions that improve security, streamline operations, and give better customer experiences.
With Arya AI's tools, Your business can use the full potential of AI to stay competitive and innovative in the rapidly evolving BFSI industry. Contact us to get started!