Deepfake is an astonishing technology that has been blurring the lines between fake and real content. It is considered a threat to the authenticity of content - image, video, or audio alike.
In July 2019, Sensity- a visual threat intelligence company, identified 14,678 deepfakes online. Eleven months later, in June 2020, that number had jumped to 49,081, an increase of 330%. Another study by The Next Web revealed that deepfake fraud attempts have increased by a whopping 3000% in 2023.
Fortunately, many deepfake detection tools can help you combat deepfakes with advanced AI technologies. In this post, we will explore the top 5 deepfake detection tools and learn how to choose the right one for your business.
What Is Deepfake Detection?
Deepfake Detection refers to identifying and verifying digital media such as pictures, videos, or audio recordings to determine whether they have been altered or produced using deep learning methods.
Fraudsters use deep learning models to create deepfakes that are AI-generated synthetic media for scamming financial institutions. They utilize advanced algorithms, such as Variational Autoencoders (VAEs) and Generative Adversarial Networks (GANs) to learn from large amounts of authentic content and produce realistic-looking images, videos, or audio that can be hard to spot.
Deepfake detection aims to identify inconsistencies in facial expressions, unnatural movements, artifacts, or anomalies in audiovisual signals and analyze them to distinguish between genuine and manipulated media. It leverages techniques such as computer vision, audio analysis, forensic analysis, machine learning, and more to recognize patterns indicative of deepfake manipulation.
By differentiating between real and fake videos or audio, deepfake detection technology helps reduce and mitigate the spread of misinformation, malicious content, harmful propaganda, and more.
Best Deepfake Detection Tools
Here are some of the best deepfake detection tools you can consider investing in.
1. Arya AI
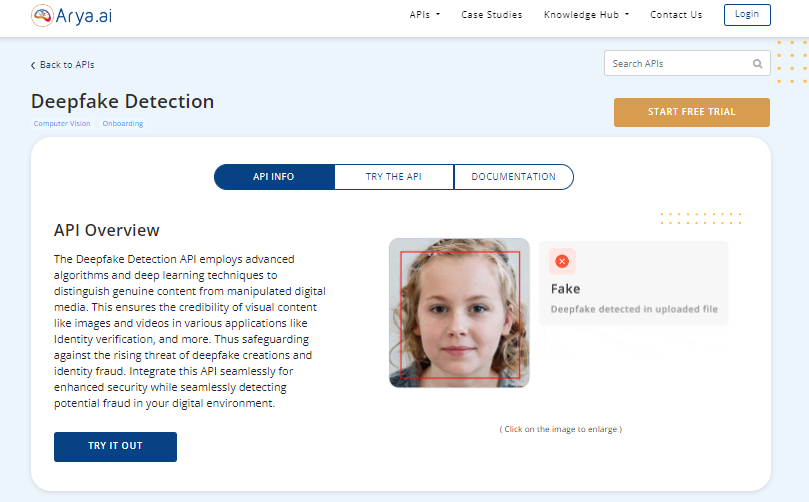
Arya AI offers an advanced Deep Fake Detection API that can be integrated seamlessly with your existing systems for efficient detection of deepfake content, such as face swaps, AI-generated images, manipulated audio, and so on. It provides an excellent tool that combines human expertise with AI analysis to conduct comprehensive assessments of digital media to identify deepfakes. Through deep learning, this AI deepfake detector can instantly detect signs of manipulation such as inconsistencies in audio and lip movements in videos, subtle gray elements in images, and so on that are generally undetectable to the human eye.
Abilities -
- Examines facial features, expressions, and movements to identify any anomalies or synthetic imagery.
- Detect and prevent fraudulent activities, such as deepfake-based scams or attempts to manipulate financial data and transactions.
- Analyze both images and videos to inspect pixel-level details, artifacts, and discrepancies in lighting, shadows, or reflections.
- Designed for easy integration into existing platforms or workflows, enabling developers to incorporate them into applications seamlessly.
Here are some top use cases for deepfake detection across different industries
Banking:
- Identity Verification: Deepfake detection can be used to enhance identity verification processes during account opening, loan applications, or transactions. By ensuring that customers' identities are genuine, banks can mitigate the risk of identity theft and fraud.
- Customer Service Authentication: Banks can employ deepfake detection to authenticate customers during interactions with customer service representatives, especially in digital channels such as video calls or chatbots, to prevent unauthorized access to accounts or sensitive information.
- Fraud Detection: Deepfake detection technology can help banks identify fraudulent activities, such as spoofing of account holders' identities in video calls or altering documentation presented for loan approvals. This aids in early detection and prevention of financial fraud.
Insurance:
- Claims Processing: Deepfake detection can be integrated into the claims processing workflow to verify the authenticity of documentation, evidence, or multimedia files submitted by policyholders. This helps insurance companies prevent fraudulent claims and reduce losses.
- Underwriting and Risk Assessment: Insurers can use deepfake detection to verify the authenticity of identity documents and medical records provided by applicants during the underwriting process. This ensures that accurate information is used for risk assessment and pricing.
- Fraudulent Injury Claims: Deepfake detection can assist insurers in validating the legitimacy of injury claims by analyzing multimedia evidence, such as photos or videos, to detect signs of manipulation or exaggeration.
Others:
- Media and Entertainment: Deepfake detection technology can be utilized by media platforms, content creators, and copyright holders to identify and mitigate the spread of manipulated content, safeguarding against unauthorized use of intellectual property and preserving the integrity of digital media.
- Elections and Politics: Deepfake detection can play a crucial role in combating misinformation and safeguarding the integrity of electoral processes by identifying and flagging manipulated videos or images used for political propaganda or fake news dissemination.
- Security and Law Enforcement: Deepfake detection can aid security agencies and law enforcement in analyzing digital evidence, such as surveillance footage or witness testimonies, to ensure their authenticity and integrity during investigations and legal proceedings.
- Education and Training: Deepfake detection technology can be integrated into educational platforms and training programs to verify the authenticity of educational materials, assessments, or certifications, thereby maintaining academic integrity and preventing cheating or credential fraud.
2. Sentinel
Sentinel is another deepfake detection tool that leverages multiple advanced technologies like temporal consistency checks, facial landmark analysis, flicker detection, and other AI-powered technologies to identify manipulated media. It analyzes multiple forms of digital media such as images, videos, audio, and more for AI forgery. When used for detecting deepfakes in digital content, it generates a comprehensive report of its results including a visualization of the manipulation.
Abilities -
- Shares a visual representation of the manipulation to help you see exactly where and how the media file has been manipulated.
- Automatically detects malicious deepfakes in real time.
- Offers automated threat remediation that responds to cyber threats automatically without requiring any manual intervention.
3. HyperVerge
HyperVerge offers a refined deepfake detection solution that employs advanced algorithms to detect deepfakes with accurate precision and high real-time confidence scores. It utilizes features like accurate image analysis, video processing, anomaly detection, facial recognition, and so on to identify instances of manipulation and deepfake-generated fraud.
Abilities -
- Proactively identifies and responds to potential deepfake threats as they emerge.
- Supports integrations with on-demand data like social media accounts.
- Has global certifications for facial recognition from NIST and iBeta.
4. Deepware
Deepware is another deepware detection tool that helps you detect and prevent deepfakes in visual and audio communication using its advanced AI engine and ML algorithms. It analyzes and detects deepfake content across images, videos, and audio recordings and assesses the authenticity of media content. You can simply input a link containing the digital media and the Deepware AI model will complete a comprehensive scan to detect any signs of manipulation and determine its authenticity.
Abilities -
- Offers real-time monitoring capabilities to automatically scan incoming media content for potential deepfakes.
- Conducts forensic analysis to examine metadata, compression signatures, and more within media files to detect traces of tampering or editing.
5. Resemble
Resemble AI uses deepfake detection technology named Resemble Detect to identify deepfake audio in real time. It is designed to distinguish between authentic and AI-generated audio and is the best tool for audio verification. It specializes in analyzing and detecting synthetic audio and providing real-time analysis.
Abilities -
- Used extensively for voice authentication to prevent unauthorized access to sensitive information.
- Compares the incoming audio recordings against the known reference voices or other authentic speech patterns for real-time voice analysis.
- Supports multiple languages and accents thereby making it suitable for work in diverse linguistic environments.
Key Features for Deepfake Detection Tools
To determine the best deepfake detection solutions, it is necessary to identify the main factors that make them effective in differentiating between genuine and fake media. Here are a few essential features you need to take into account.
1. Algorithmic Analysis
Most deepfake detection tools rely on sophisticated algorithms that depend on machine learning and computer vision to study and analyze audio and visual media files. Such algorithms review your files for inconsistencies, irregularities, or artifacts that could signify alterations or manipulation.
For example, it may detect disparities in facial expressions, unnatural movements, or abnormal audio patterns in a video clip. Hence, your deepfake detection tool needs to be trained on diverse datasets - both authentic and manipulated media to teach it to recognize patterns related to deepfake manipulation. Thus enabling them to identify dubious content accurately.
2. Visual Artifacts Analysis
Deepfake detection tools rely on visual artifact analysis to detect deepfakes, which examine the visual characteristics and irregularities found in media files that could indicate manipulation. This includes distortions, inconsistencies, or anomalies in the visual content, like unnatural facial expressions, mismatched lighting or shadows, or irregular pixel patterns.
It also helps you detect synthetic elements by checking for color variations, spatial relations within an image or video frame where none should exist naturally, etc. For example, it can accurately detect face swapping, where a customer's facial identity is replaced with someone else's convincingly. Similarly, by comparing the temporal coherence of visual elements, you can spot areas of the media file where manipulation may have occurred.
Deepfake detection tools can also give you actionable information to verify or debunk the authenticity of media content.
3. Facial Biometrics
The facial biometrics feature helps you verify your customer's identity in digital media using advanced facial recognition algorithms and biometric analysis methods. By comparing facial features, such as proportions, landmarks, textures, and more against the standard biometric or reference images; you can determine whether a face has been modified or fabricated by deepfake programs. Besides, facial biometrics analyzes the consistency of facial characteristics across different frames within the media file that may be strongly indicative of deepfake manipulation. It offers valuable evidence for distinguishing between original video footage from those that were tampered with.
4. Audio-Visual Synchronization
The audio-visual synchronization feature analyzes the alignment between the audio and video elements within the media files, especially videos to detect manipulation. By examining lip movements, facial liveliness, spoken words, and other elements, you can determine the naturalness and consistency of audio-visual synchronization. It identifies the relationship between audio and visual cues, such as lip movements not matching the spoken words or weird facial expressions to detect manipulated media content. It flags audio and visual elements that are out of sync and provides valuable insights into the authenticity of media files.
5. Contextual Analysis
Contextual analysis helps you evaluate the context surrounding the media content to assess its credibility and authenticity. For example, it looks at where a piece of content comes from or if its storyline matches with what is happening around it. It also examines the metadata to determine whether timestamps and provenance information are consistent with expected origins or not. Conducting contextual analysis helps you evaluate source reliability by considering various factors that may increase chances for manipulation. It gives out a more comprehensive understanding of media content, thereby helping you make informed decisions about its authenticity.
6. Texture Analysis
Texture analysis conducts a detailed examination of very fine features or details in digital media like images and videos; patterns of textures, gradients as well as other variations. It can detect faults by checking how well textures match with each other across an image or video, where they should have been consistent according to natural laws. It is also possible for them to detect abnormalities among regions that were expected to be the same but they appear not similar due to manipulation.
Texture analysis differentiates between real and fake by identifying areas with unnatural color changes or surface roughness that would not occur normally. This is effective when dealing with minimal modifications made on files thus making it more reliable in finding synthetic components used while creating them too.
7. Forensic Analysis
Deepfake detection tools must utilize forensic analysis techniques to assess metadata, compression signatures, and other digital footprints within the media files. This helps you identify inconsistencies with compression artifacts, such as traces of editing or tampering, timestamp discrepancies, poor recording quality, or more used in the manipulation process. Forensic analysis helps to boost the trustworthiness of deepfake detections as it digs into the digital marks left behind when modifying a media file and serves as the best proof for manipulation.
8. Real-time Monitoring
Most deepfake detection tools offer real-time monitoring capabilities that allow you to automatically scan incoming media content for signs of manipulation as and when they are shared or uploaded. This real-time monitoring helps you to take quick action against harmful deepfakes that could otherwise have an adverse impact on your business. These tools can help you tackle misinformation through proactive monitoring and flagging suspicious content for timely resolution.
Choosing the Right Tool for Deepfake Fraud Prevention
Deepfake detection tools play a crucial role in identifying and mitigating manipulated media content. These tools detect and reduce the impact of manipulated media content by marking out deepfakes.
Selecting an effective deepfake detection tool helps preserve trust and credibility in digital media, which flags false or misleading content. Mitigating the risks associated with deepfake-generated AI fraud helps protect individuals, organizations, and brands from reputational damage and potential legal liabilities resulting from the spread of manipulated media.
To enhance overall cybersecurity, you should invest in deepfake fraud prevention tools and prioritize the prevention of manipulated media generation. This will help you mitigate the risks of social engineering attacks, identity theft, and other fraudulent activities facilitated by deepfake manipulation. Implementing robust deepfake detection measures also helps organizations comply with regulatory requirements related to data protection, consumer privacy, and content moderation in digital environments.
That being said, let us understand how to choose the right tool for deepfake fraud prevention and safeguard your business against the use of manipulated media. Here is a short list outlining the crucial considerations.
- Detection Precision - Evaluate the system's accuracy in identifying deepfakes across different media types, such as images, video clips, or sound recordings.
- Sophistication of Algorithm - Determine the complexity of algorithms the chosen software employs to recognize altered reality. This is necessary to understand if they can withstand even more advanced deepfaking techniques.
- Real-Time Monitoring Capability - Ensure that the chosen software can automatically scan, detect, and flag suspicious items immediately upon upload or as and when shared with you.
- User Interface Design - Opt for a tool with an easy-to-use interface that is intuitive enough even for beginners without much technical knowledge. This will ensure it will be fully utilized by experts across all functions.
- Flexibility in Integration - Ensure that the chosen software integrates seamlessly into existing structures and operations. Check whether it allows easy sharing of data between different security systems within an organization or content management platforms.
Conclusion
Efficient deepfake detection tools help safeguard your business against manipulated media content. By utilizing advanced AI technologies and offering real-time monitoring, these deepfake fraud detection tools address the complex challenges posed by deepfake fraud and mitigate the risks associated with them.
Arya AI offers an excellent deepfake detection API that leverages facial biometrics, contextual analysis, textual analysis, and more for analyzing media files and identifying instances of manipulated content. It is a robust deepfake detection solution that helps you safeguard the authenticity of digital media. Connect with our team to learn how to integrate our APIs to guarantee the authenticity of your media content.